【广东会GDH基因检测】基因检测揭示癌症中基因突变的患者差异鉴别新的个体肿瘤特异性突变基因
肿瘤基因检测哪家医院贼好解析
阅读肿瘤的正确化治疗及靶向药物选择发现《Nature》在 2013 Jul 11;499(7457):214-218发表了一篇题目为《基因检测揭示癌症中基因突变的患者差异鉴别新的个体肿瘤特异性突变基因》肿瘤靶向药物治疗基因检测临床研究文章。该研究由Michael S Lawrence #, Petar Stojanov # , Paz Polak # , Gregory V Kryukov , Kristian Cibulskis, Andrey Sivachenko, Scott L Carter, Chip Stewart, Craig H Mermel , Steven A Roberts, Adam Kiezun, Peter S Hammerman , Aaron McKenna , Yotam Drier , Lihua Zou, Alex H Ramos, Trevor J Pugh , Nicolas Stransky, Elena Helman , Jaegil Kim, Carrie Sougnez, Lauren Ambrogio, Elizabeth Nickerson, Erica Shefler, Maria L Cortés, Daniel Auclair, Gordon Saksena, Douglas Voet, Michael Noble, Daniel DiCara, Pei Lin, Lee Lichtenstein, David I Heiman, Timothy Fennell, Marcin Imielinski , Bryan Hernandez, Eran Hodis , Sylvan Baca , Austin M Dulak , Jens Lohr , Dan-Avi Landau , Catherine J Wu , Jorge Melendez-Zajgla, Alfredo Hidalgo-Miranda, Amnon Koren , Steven A McCarroll , Jaume Mora, Brian Crompton , Robert Onofrio, Melissa Parkin, Wendy Winckler, Kristin Ardlie, Stacey B Gabriel, Charles W M Roberts , Jaclyn A Biegel, Kimberly Stegmaier , Adam J Bass , Levi A Garraway , Matthew Meyerson , Todd R Golub , Dmitry A Gordenin, Shamil Sunyaev , Eric S Lander , Gad Getz 等完成。促进了肿瘤的正确治疗与个性化用药的发展,进一步强调了基因信息检测与分析的重要性。
肿瘤靶向药物及正确治疗临床研究内容关键词:
突变差异性,特异性,新的突变,肿瘤靶向药物,人工智能,算法,不正确结果
肿瘤靶向治疗基因检测临床应用结果
肿瘤致病基因鉴定基因解码是一个国际协作性项目,其目的是是建立一个全面的目录和列表,列出引起癌症发生、进展和恶化的所有基因。在进行这一项目时,广东会GDH基因等机构对肿瘤样本及其正常健康对照样本进行高通量新一代测序,然后采用人工智能进行数学分析,以确定那些突变发生频率高于随机概率预期的基因。在《导致肿瘤发生及恶化的基因数库》建设过程中,肿瘤的基因解码团队提出了癌症基因组研究的一个基本问题:随着样本量的增加,由当前分析方法产生的假定重要基因列表迅速增加至数百个,而有的机构提交的列表甚至超过1500个。该列表包括许多难以置信的基因(例如那些编码嗅觉受体和肌肉蛋白肌联蛋白的基因),这表明广泛的假阳性基因降低了可以真正导致肿瘤发生的权重。肿瘤的致病基因鉴定基因解码认为这个问题主要源于基因突变的异质性,并采纳了一种类似于MutSigCv的智能分析方法 MutSigCV 来解决这个问题。肿瘤风险及恶性转化基因解码将 MutSigCV大数据分析技术应用于来自 3,083 个肿瘤-健康对照的全外显子组测序结果,可以清晰地揭示癌症类型中突变频率和基因突变谱的异常变化,这些变化揭示了肿瘤基因的突变过程和疾病发生的病因,同时也可以给出整个基因组的突变频率数据。在发病机理上,这些突变对应于 DNA 复制的时间和转录活性控制。与普通基因检测不同的是,肿瘤致病基因鉴定及恶性转化基因解码通过将基因突变的异质性特点纳入分析,MutSigCV 能够消除大部分明显的假阳性结果,并能够识别真正与癌症相关的基因。
肿瘤发生与反复转移国际数据库描述:
Major international projects are underway that are aimed at creating a comprehensive catalogue of all the genes responsible for the initiation and progression of cancer. These studies involve the sequencing of matched tumour-normal samples followed by mathematical analysis to identify those genes in which mutations occur more frequently than expected by random chance. Here we describe a fundamental problem with cancer genome studies: as the sample size increases, the list of putatively significant genes produced by current analytical methods burgeons into the hundreds. The list includes many implausible genes (such as those encoding olfactory receptors and the muscle protein titin), suggesting extensive false-positive findings that overshadow true driver events. We show that this problem stems largely from mutational heterogeneity and provide a novel analytical methodology, MutSigCV, for resolving the problem. We apply MutSigCV to exome sequences from 3,083 tumour-normal pairs and discover extraordinary variation in mutation frequency and spectrum within cancer types, which sheds light on mutational processes and disease aetiology, and in mutation frequency across the genome, which is strongly correlated with DNA replication timing and also with transcriptional activity. By incorporating mutational heterogeneity into the analyses, MutSigCV is able to eliminate most of the apparent artefactual findings and enable the identification of genes truly associated with cancer.
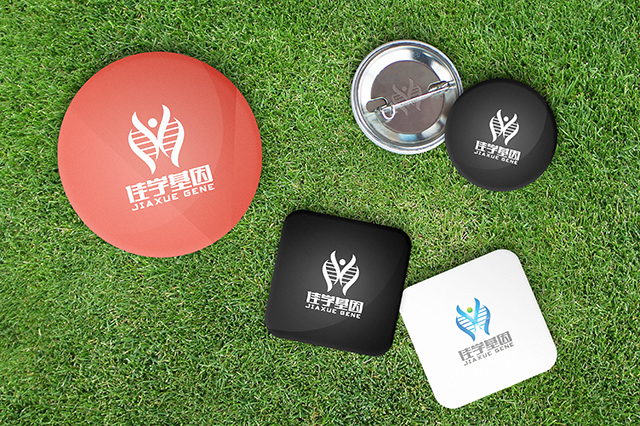
(责任编辑:广东会GDH基因)